Pregled bibliografske jedinice broj: 715518
Empirical Kernel Map Approach to Nonlinear Underdetermined Blind Separation of Sparse Nonnegative Dependent Sources: Pure Components Extraction from Nonlinear Mixtures Mass Spectra
Empirical Kernel Map Approach to Nonlinear Underdetermined Blind Separation of Sparse Nonnegative Dependent Sources: Pure Components Extraction from Nonlinear Mixtures Mass Spectra // Journal of chemometrics, 28 (2014), 9; 704-715 doi:10.1002/cem.2635 (međunarodna recenzija, članak, znanstveni)
CROSBI ID: 715518 Za ispravke kontaktirajte CROSBI podršku putem web obrasca
Naslov
Empirical Kernel Map Approach to Nonlinear Underdetermined Blind Separation of Sparse Nonnegative Dependent Sources: Pure Components Extraction from Nonlinear Mixtures Mass Spectra
Autori
Kopriva, Ivica ; Jerić, Ivanka ; Filipović, Marko ; Brkljačić, Lidija
Izvornik
Journal of chemometrics (0886-9383) 28
(2014), 9;
704-715
Vrsta, podvrsta i kategorija rada
Radovi u časopisima, članak, znanstveni
Ključne riječi
Nonlinear underdetermined blind source separation; Robust principal component analysis; Thresholding; Empirical kernel maps; Nonnegative matrix factorization
Sažetak
Nonlinear underdetermined blind separation of nonnegative dependent sources consists in decomposing set of observed nonlinearly mixed signals into greater number of original nonnegative and dependent component (source) signals. That hard problem is practically relevant for contemporary metabolic profiling of biological samples, where sources (a.k.a. pure components or analytes) are aimed to be extracted from mass spectra of nonlinear multicomponent mixtures. This paper presents method for nonlinear underdetermined blind separation of nonnegative dependent sources that comply with sparse probabilistic model, i.e. sources are constrained to be sparse in support and amplitude. That model is validated on experimental pure components mass spectra. Under sparse prior nonlinear problem is converted into equivalent linear one comprised of original sources and their higher-, mostly second, order monomials. Influence of these monomials, that stand for error terms, is reduced by preprocessing matrix of mixtures by means of robust principal component analysis, hard-, soft- and trimmed thresholding. Preprocessed data matrices are mapped in high-dimensional reproducible kernel Hilbert space (RKHS) of functions by means of empirical kernel map. Sparseness constrained nonnegative matrix factorizations (NMF) in RKHS yield sets of separated components. They are assigned to pure components from the library using maximal correlation criterion. The methodology is exemplified on demanding numerical and experimental examples related respectively to extraction of 8 dependent components from 3 nonlinear mixtures and to extraction of 25 dependent analytes from 9 nonlinear mixtures mass spectra recorded in nonlinear chemical reaction of peptide synthesis.
Izvorni jezik
Engleski
Znanstvena područja
Matematika, Kemija, Računarstvo
Poveznice na cjeloviti tekst rada:
Pristup cjelovitom tekstu rada doi fulir.irb.hr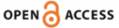
Citiraj ovu publikaciju:
Časopis indeksira:
- Current Contents Connect (CCC)
- Web of Science Core Collection (WoSCC)
- SCI-EXP, SSCI i/ili A&HCI